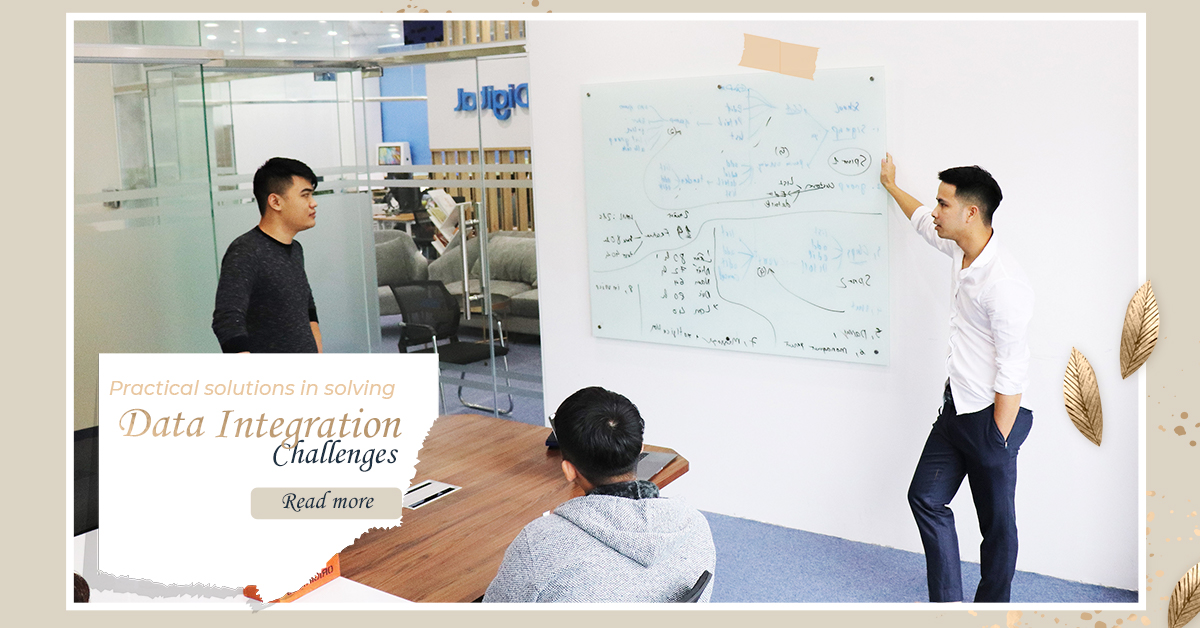
The development of big data and blockchain give birth to data integration challenges. With tremendous data generated daily, companies need solutions to tackle it.
The modern world is the world of sharing and connecting that the root of this concept refers to the capacity to freely sharing their media or data. Whereas most of those shared data is not follow the same format, it is seemingly impossible to consolidate them. Additionally, there is no official standard for data collections that companies currently leverage their custom software system in storing and processing data. Accordingly, to data integration, those companies need to scarify their secret. Obviously, there is limited form willing to do it.
However, dada might not be performing their authentic roles when sitting separately. We live in the hyper-connection world when every minor change in a conner makes the butterfly effect to other space. The whole industries take full advantage of data only if it offers accessibility and data integration solutions. In this article, we discuss the data integration challenges and how they can be tackled.
Overview of data integration services
Basically, data integration explains the process that data analysis companies combine and structure raw data from diverse sources into a single view form, which is ready to analyze. Undoubtedly, the consolidation of data from disparate sources needs an intermediary to ensure fairness to participated parties.
In this case, data integration services have been developed to control the process of sharing and integrating data. They currently execute some software development projects in building data integration software as a service or a platform to automatically route raw data into the system.
The simple model of the data integration process consists of four fundamental steps:
Step 1: Data extraction: raw datasets are extracted from various data sources
Step 2: Data transformation: extracted data would be gathered together into a meaningful data chain.
Step 3: Data Cleansing: transformed data would be harmonized, removing errors or junks to keep the data in a typical form.
Step 4: Loading integrated data: Datasets after cleansing need to be arranged and loaded to store in constructed database
Recently, businesses are familiar with a new term of big data integration, which mentions management and data control process of massive data volume, which is transformed into a single framework. Obviously, the amount of data loading from the regular data integration process tends to be a minor piece in big data integration. From the rapid growth of the Internet of Thing (IoT), embracing in several industries, big data integration come with scalability and high performance.
Data integration applications focus on loading integrated data for future trend prediction and analyze the business performance. Accordingly, the massive data sharing daily, it’s time for real-time big data integration.
Data integration technique
In practice, due to the fact that lack of a data integration framework to follow, different data integration services applied different data integration techniques. Fortunately, we can generate five fundamental tools to consolidate datasets:
- Common user interface: specialize process of integrating data manually, applied for unified data within a limitation in scale. A common user interface requires a significant effort of data integration staff.
- Data integration by a set of applications: which consist of application development to implement the integration process, follow the same efforts.
- Data integration by middleware: plays a role as the connection to gather data from partners.
- Physical data integration: needs custom system development to copy and store data from sources to an independent data warehouse.
Cloud data integration
As mention above, the future of data refers to real-time data integration. To deliver its concept, data architects need software development solutions to streamline various sides in the data chain, including system, applications, database, and technology environments. Accordingly, it keeps the accessibility to real-time exchange and combination data from various inceptions. Hence, cloud data integration was born.
With the popularity of SaaS (software as a service) companies, cloud data integration solution is believed to enhance the hyperconnectivity and visibility of data silos. It provides accessibility to parties into a cloud-based system, which eliminates the challenges of device difference.
Data integration challenges
Disadvantages of data integration
One of the most significant disadvantages of date integration refers to the concern regarding data security. From the data owners’ perspective, adopting data integration means they take the challenges of insecure data. Since the data integration process come up with series of step with the participation of several parties, they might lose the data confidentiality. In fact, the data breach concern reaches the top challenges regarding why companies are afraid to join the data chain.
The profound application of data integration in the business context tends to be the capacity of modeling metrics into reports. Via reports and analyses, business owners expect to conclude with decisions and strategies. However, executing data integration would steal the ability of data monitoring from companies.
Adopting data integration is costly, obviously. For software development companies, concerning data integration challenges should find a way to optimize the cost for developing, using, and maintaining data integration solutions. Undeniably, the amount of cost for those above tasks is enormous that not all companies can afford.
Data integration challenges in data mining
The process of data mining indicates the effort of extracting valued information or gathering predictions from raw data. Accordingly, data integration is believed to decide the quality of data mining that any distorted data would lead the information extraction to failure. In fact, suffering data integration challenges cause data redundancy and data conflicts.
Data redundancy occurred when data input contains inconsistent attributes. It causes data fields to be dependent and merging each other. In this case, data architects need to do further analysis to detect data redundancy before executing data integration.
Data conflicts tend to be a troublesome question in designing a data integration system. In which some of the data attributes might be printed in diriment forms from the different data sets. Additionally, the problem became more serious when the data collected being conflicted with the res world entity. In this case, dataset or data loading has minimal contribution in data mining as they do not tell the truth.
Ambiguous defining among datasets
For manual data integration, it is commonly struggling with errors in formatting and validation data. The failure occurred when an ambiguous definition was made, which cause misunderstanding between companies and data integration teams. Hence, a shared dictionary of data definition is necessary and fundamental before executing any data integration process.
A common dictionary is supported by two elements, including data governance and data stewardship. The data governance controls the procedures of data integration strategies. Additionally, data stewardship focuses on having an individual who is responsible for covering all contributors in data integration strategies.
Data integration solution
- Collaboration: in the business side, the most significant challenges of data integration came from the imbalance benefits for each party. Many countries are not willing to share their data and technology due to the doubt of disclosing trade secrets. At this point, close collaboration is believed to be an effective solution. Once benefit-sharing gets verified to all vendors, the data ownership would end up with the better operation of data integration systems.
- Multi-directional data synchronization: the middlemen’s participation in data integration is believed to a longer time of processing, while it is also blamed for deviation in data transmission. Accordingly, companies should consider the smart data integration platform or other solution that allows multi-directional data synchronization. In which they can reduce the contribution of the middleman.
- Leveraging data transformation tools would help enterprises dealing with data, which is not formatted correctly. It allows automated adjustment inside data based to minimize the potential errors in the data transformation pipeline.
- To tackle the data integration challenges of junk data or poor-quality data, experts believed in the data validation approach. Before entering the system, data should follow a comprehensive check for validation to detect errors.
The prominence of the IT sector has grown dramatically. An IT development company can assist you in constructing unique services to optimize business processes.
Read
Firms offer custom software application development services such as CRM, ERP systems, and other lucrative software solutions. Read benefits for businesses.
Read
Having a mobile app is becoming a given for companies of all sizes. The first step to making an app is to understand how much does it cost to make an app?
Read
Comments